Biological Data Analysis
Biological system can be regarded as a high-dimensional dynamical system with a complex structure. There are only a few variables that can be observed externally in an uncertain and noisy environment. In many cases, there is a large discrepancy between what we want to know and what we can measure.
The key issues are how to model the generation mechanism of observable data in a way that concisely models the essential parts of the structure we want to know, and how to efficiently and accurately estimate unknown parameters.
Estimating Neural Connectivity from Spike Trains
Estimating neural connections is a fundamental problem in order to understand functions of biological systems and learning mechanisms. Although electrode recording is a rather classical tool, recent development allows us to measure activities of multiple neurons simultaneously and continuously. For multiple spike train analysis, we propose a method to solve
- pseudo correlation caused by higher-order effect and dynamics,
- influence from unobserved neurons,
- directional excitatory/inhibitory connections.
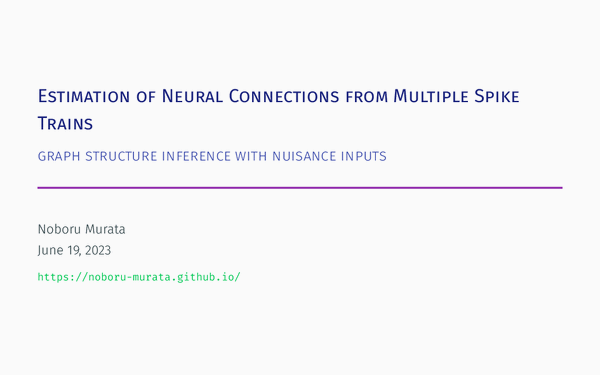
Iwasaki, T., Hino, H., Tatsuno, M., Akaho, S., Murata, N.: “Estimation of neural connections from partially observed neural spikes”, Neural Networks, Volume 108, December 2018, Pages 172-191. https://doi.org/10.1016/j.neunet.2018.07.019
related works
Nagayama, M., Aritake, T., Hino, H., Kanda, T., Miyazaki, T., Yanagisawa, M., Akaho, S., Murata, N.: “Detecting cell assemblies by NMF-based clustering from calcium imaging data”, Neural Networks, Volume 149, May 2022, Pages 29-39. https://doi.org/10.1016/j.neunet.2022.01.023
Biologically Inspired Image Super-Resolution
It is said that the microsaccade (fixation micro-motion of our eyeballs) has various functions, and one of them is to acquire super-resolution information by causing misalignment in the acquired images. Based on this idea, we proposed an algorithm for super-resolution by aligning small regions (patches) of several consecutive images, and decomposing a low-resolution image and recombining a high-resolution image in each patch by sparse coding.
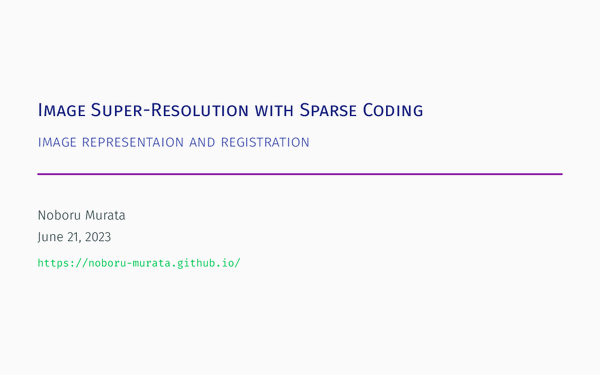
Kato, T., Hino, H., Murata, N.: “Multi-frame image super resolution based on sparse coding”, Neural Networks, Volume 66, June 2015, Pages 64–78. https://doi.org/10.1016/j.neunet.2015.02.009
Kato, T., Hino, H., Murata, N.: “Double sparsity for multi-frame super resolution”, Neurocomputing, Volume 240, May 2017, Pages 115–126. https://doi.org/10.1016/j.neucom.2017.02.043
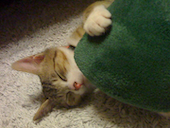